EHR的問題,我們搜遍了碩博士論文和台灣出版的書籍,推薦Scariati, Paula寫的 Ehr Governance: A Practical Guide to User Centric, Consensus Driven Optimization 和Scariati, Paula的 Ehr Governance: A Practical Guide to User Centric, Consensus Driven Optimization都 可以從中找到所需的評價。
另外網站Switch EHR vendors or update Identity Providers - Apple ...也說明:If you're a program administrator supporting Health Records on iPhone, contact Apple before switching EHR vendors or updating IDPs.
這兩本書分別來自 和所出版 。
國立中正大學 資訊管理系研究所 胡雅涵、李珮如所指導 宋昇峯的 以監督式機器學習探討電子病歷中非結構化資料對早期預測中風後功能復原後果之價值 (2021),提出EHR關鍵因素是什麼,來自於急性缺血性中風、電子病歷、功能復原後果、機器學習、敘述式臨床紀錄、自然語言處理、風險模型、預測。
而第二篇論文國立清華大學 資訊工程學系 許聞廉、陳宜欣所指導 王鳳鐸的 詞嵌入增強之統計準則式方法於病例去識別化之應用 (2021),提出因為有 去識別化、語言模板、深度學習、語意編碼、實體命名識別、詞嵌入的重點而找出了 EHR的解答。
最後網站Electronic Health Record (EHR) Resources | AAN - American ...則補充:Access the AAN's Electronic Health Record (EHR) resources for information, templates, and educational programming, to benefit your practice and patients.
Ehr Governance: A Practical Guide to User Centric, Consensus Driven Optimization
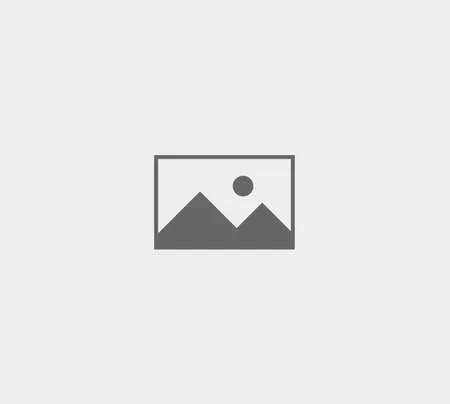
為了解決EHR 的問題,作者Scariati, Paula 這樣論述:
Dr. Paula Scariati is a board-certified preventive medicine and public health physician with a subspecialty certification in clinical informatics. Paula is currently a Medical Director of Health Informatics at CommonSpirit Health, the largest non-for-profit healthcare system in the U.S. During her c
areer in informatics, Dr. Scariati helped deploy 13 hospitals on Cerner Millennium to HIMSS Level 6 in 27 months. A former CMIO at a 235-community hospital, Paula is a CDC-trained epidemiologist with expertise in research models and methods, evidence-based medicine, public health, health policy, and
quality improvement. She’s authored 13 peer reviewed publications, is an award-winning medical school instructor, software developer and seasoned national speaker. She has nineteen years of clinical experience and is also an ASQ certified Lean Six Sigma Green Belt.
EHR進入發燒排行的影片
以監督式機器學習探討電子病歷中非結構化資料對早期預測中風後功能復原後果之價值
為了解決EHR 的問題,作者宋昇峯 這樣論述:
中風是導致成人殘障的重要原因,中風功能復原後果的精準預測,能協助病人及家屬及早準備後續照顧事宜,衛生政策制定者也能依此預測結果適切規劃人力與資源,以投入中風病人的急性後期與中長期照護。目前的中風功能復原後果預測模型皆是以結構化資料建立,甚至最新使用數據驅動方式發展的機器學習預測模型依然是以結構化資料為主。相對的,照顧病人所製作的大量敘述式病歷文字紀錄,即非結構化資料,反而甚少被使用。因此,本研究的目的,即是使用監督式機器學習來探討非結構化臨床文字紀錄於急性缺血性中風後之初期預測功能復原後果之應用價值。在6176位2007年10月至2019年12月間因急性缺血性中風住院之病人中,共3847位病
人符合本研究之收案/排除條件。我們使用自然語言處理,萃取出住院初期之醫師紀錄及放射報告中之臨床文字紀錄,並且實驗了不同文字模型與機器學習演算法之組合,來建構中風功能復原後果的預測模型。實驗發現使用醫師紀錄時,操作特徵曲線下面積為0.782至0.805,而使用放射報告時,曲線下面積為0.718至0.730。使用醫師紀錄時,最好的組合為詞頻-倒文件頻加上羅吉斯迴歸,而使用放射報告時,最好之組合為基于轉換器的雙向編碼器表示技術加上支持向量機。這些基於純文字的機器學習預測模型並無法勝過傳統的風險模型,這些傳統模型的曲線下面積為0.811至0.841。然而,不管是以曲線下面積、重分類淨改善指標、或整合式
區辨改善指標來評估,臨床文字紀錄中的資訊的確可以增強傳統風險模型的預測效能。本研究之結論為,電子病歷中的非結構化文字經過自然語言處理後,不僅可以成為另類預測中風功能復原後果的工具,更可以增強傳統風險模型的預測效能。透過演算法來自動擷取並整合分析結構化與非結構化資料,將能提供醫師更好的決策支援。
Ehr Governance: A Practical Guide to User Centric, Consensus Driven Optimization
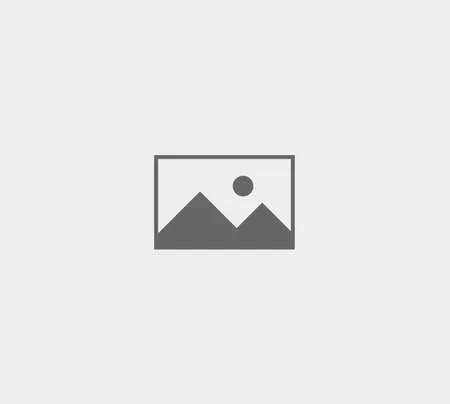
為了解決EHR 的問題,作者Scariati, Paula 這樣論述:
Dr. Paula Scariati is a board-certified preventive medicine and public health physician with a subspecialty certification in clinical informatics. Paula is currently a Medical Director of Health Informatics at CommonSpirit Health, the largest non-for-profit healthcare system in the U.S. During her c
areer in informatics, Dr. Scariati helped deploy 13 hospitals on Cerner Millennium to HIMSS Level 6 in 27 months. A former CMIO at a 235-community hospital, Paula is a CDC-trained epidemiologist with expertise in research models and methods, evidence-based medicine, public health, health policy, and
quality improvement. She’s authored 13 peer reviewed publications, is an award-winning medical school instructor, software developer and seasoned national speaker. She has nineteen years of clinical experience and is also an ASQ certified Lean Six Sigma Green Belt.
詞嵌入增強之統計準則式方法於病例去識別化之應用
為了解決EHR 的問題,作者王鳳鐸 這樣論述:
現今網路的發展下,要取得大量的訓練資料,並非是一件難事,但如果是要取得一些會涉及到個人資訊的資料 (如:合約、病例),就會衍生出相關的法律問題,舉例來說健保署於2016年時,就有因公布健保資料,導致發生行事訴訟。因而,要在這些資料上,去衍生出模型或是應用,往往需要把含有個人訊息的資料(如:人名,身分證字號,地址,電話,年齡…等等)去做改寫或是替換。例如: 可以把原年齡都往上增加十歲,假設原年齡為20歲,便可改成30歲。便能保護原資料者的個人訊息 ,同時在使用這些資料時 ,不會破壞掉原資料的架構與內容。本研究提出以語言模板(Linguistic Patten)為基礎,結合深度學習的做法,建構命
名實體識別(Named Entity Recognition)模型,相較於現今實驗大多以深度學習為主,此方法能有較好的可讀性且容易維護,並同時兼具高準確率的優點。我們將以此研究方法建構病例去識別化模型,同時將模型與現今實體識別模型去做比較。
EHR的網路口碑排行榜
-
#1.Electronic Health Records (EHR) Research Group | LSHTM
A diverse team capitalising on research opportunities offered by routinely collected data, bringing methodological rigour to provide real-world evidence for ... 於 www.lshtm.ac.uk -
#2.EHR供應商在全球各地市場概況- DIGITIMES 智慧應用
世界各地對於電子健康紀錄(Electronic Health Record;EHR)服務供應商的滿意度不一,KLAS調查34個國家215個組織,訪問對EHS供應商的感想。 於 www.digitimes.com.tw -
#3.Switch EHR vendors or update Identity Providers - Apple ...
If you're a program administrator supporting Health Records on iPhone, contact Apple before switching EHR vendors or updating IDPs. 於 support.apple.com -
#4.Electronic Health Record (EHR) Resources | AAN - American ...
Access the AAN's Electronic Health Record (EHR) resources for information, templates, and educational programming, to benefit your practice and patients. 於 www.aan.com -
#5.Toward a smarter electronic health record | MIT News
Electronic health records have been widely adopted with the hope they would save time and improve the quality of patient care. 於 news.mit.edu -
#6.Electronic Health Record (EHR) Information
An Electronic Health Record (EHR) is the health record of an individual with current information that can be used by health care providers to provide high ... 於 www.health.state.mn.us -
#7.EHR Software Application (Electronic Health Records) | Kareo
Improve the quality of care that you provide with award winning Kareo EHR Software for e-prescribing, managing electronic superbills and updating electronic ... 於 www.kareo.com -
#8.Ascension, Google Expand Pilot of EHR Search Tool for ...
Leaders at Google and the St. Louis, Mo.-based Ascension say they are expanding the clinical pilot of an electronic health record (EHR) ... 於 www.hcinnovationgroup.com -
#9.Electronic Health Record Software| EHR & EMR Solutions
Netsmart's electronic health records (EHRs) offer workflows and functionality that are purpose-built for human services and post-acute care providers, ... 於 www.ntst.com -
#10.Definition of Electronic Health Record (EHR) - Gartner
The EHR provides support for all activities and processes involved in the delivery of clinical care. The definition of an EHR system limits its scope to the ... 於 www.gartner.com -
#11.EHRIntelligence: EHR optimization, interoperability, and ...
Electronic health record (EHR/EMR) news. Stay up to date with EHR News, EHR Replacement, HIE, EHR Incentive guidelines and MACRA. 於 ehrintelligence.com -
#12.Electronic Health Records Software | ADS - Advanced Data ...
Our built-in Medics FlowText makes the MedicsCloud EHR voice navigable. When using a system such as Dragon Medical One, FlowText intelligently inserts data ... 於 www.adsc.com -
#13.Using Electronic Health Records for Population ... - PubMed
The use and functionality of electronic health records (EHRs) have increased rapidly in the past decade. Although the primary purpose of EHRs is clinical, ... 於 pubmed.ncbi.nlm.nih.gov -
#14.Electronic Health Record (EHR)
Converting to an electronic health record system can help retrieval time and accessibility. Learn of the benefits by converting patient medical records. 於 www.recordnations.com -
#15.Practice EHR | EMR & EHR Software for Medical Practices
Practice EHR is a cloud-based medical software solution that handles and streamlines tasks—allowing for a simplified workflow and increased productivity. 於 www.practiceehr.com -
#16.Electronic Health Records - State of NJ
Electronic Health Records (EHRs) are digital versions of patients' charts that are available to health care providers wherever and whenever they are needed. 於 www.nj.gov -
#17.The Federal Government Has Put Billions into Promoting ...
For physicians willing to adopt EHRs, the financial incentives offered by the ... That is, transitioning to an EHR requires a significant time investment, ... 於 www.commonwealthfund.org -
#18.VA EHR Modernization - Home
The Department of Veterans Affairs is committed to providing seamless care for Veterans, including access to a comprehensive electronic health record. 於 www.ehrm.va.gov -
#19.EHR Association |
The HIMSS Electronic Health Record (EHR) Association is a trade association ... widespread adoption of EHRs will help improve the quality of patient care as ... 於 www.ehra.org -
#20.EHR National Symposium | Stanford Medicine
Thank you for joining Stanford Medicine for its second EHR National ... and policy discussed the future of electronic health record systems and their ... 於 med.stanford.edu -
#21.Electronic Health Record Software (EHR) for Fire - ESO
Electronic Health Record Software (EHR). Easy-to-produce quality EMS clinical documentation. Yet it's so advanced, it actually gives you back more data than ... 於 www.eso.com -
#22.EMR or EHR? What's the difference? - Paubox
An EHR is more than a digital version of a patient's paper chart. While it does include medical information, it could also include billing ... 於 www.paubox.com -
#23.e-HR 玉山人園地
e-HR 玉山人園地登入. 請選擇服務單位, 玉山銀行, 玉山金控, 玉山證券, 玉山創投, 玉山投顧. 玉山銀行人力資源處服務專線. (02)2175-1313分機8701. 於 ehr.esunbank.com.tw -
#24.Elation EHR Software for Clinic-First Practice Management
Certified, cloud-based electronic health records (EHR). Spend your time on things that matter and focus on phenomenal care. Quick training, and 24/7 ... 於 www.elationhealth.com -
#25.EHR | iThome
過去使用機器學習預測加護病房病患的情況,屬於單任務學習,也就是僅能預測特定不良情況,而現在Google採用SeqSNR演算法,更能掌握病人整體狀態給出綜合預測. 於 www.ithome.com.tw -
#26.electronic health record | Description, Implementation, & Issues
The technical infrastructure of electronic health records (EHRs) varies according to the needs of the health care provider or other entity using the system ... 於 www.britannica.com -
#27.Electronic Health Records (EHR) and Clinical Decision Support
Electronic Health Records (EHR) and Clinical Decision Support · The EHR eliminates legibility problems with handwritten records (although may introduce errors ... 於 www.msdmanuals.com -
#28.Benefits of Electronic Health Records
Healthcare providers can use EHR in an emergency situation to get a more accurate picture of a patient's medical history more quickly than with ... 於 www.usfhealthonline.com -
#29.EMR vs EHR – What is the Difference? - NextGen Healthcare
By contrast, an EHR contains the patient's records from multiple doctors and provides a more holistic, long-term view of a patient's health. It includes their ... 於 www.nextgen.com -
#30.Electronic health records (EHR) - TELUS
Learn about electronic health records (EHR) and the benefits of using our EHR solutions, which centralize patient information and help enhance efficiency ... 於 www.telus.com -
#31.Certified EHR Technology - Georgia Department of ...
To efficiently capture and share patient data, health care providers need an Electronic Health Record (EHR) that stores data in a structured format. 於 dch.georgia.gov -
#32.The EHR Is Dead. Long Live The EHR Platform (1 Of 2) - Forbes
In an era that predated electronic health records (EHRs) policies and mandates, Gingrich was part of a bipartisan consensus that advocated ... 於 www.forbes.com -
#33.Electronic Health Records (EHR) Integration | Mass.gov
Integrating MassPAT data within an Electronic Health Record (EHR) will provide a streamlined clinical workflow for providers. The integration will eliminate ... 於 www.mass.gov -
#34.EHR-O - Echaintool
圖示, 商品型號, 在庫, 寸法(mm), 無頭螺絲, 螺絲. D1, D, L. EHR-0632-O, 6, 32, 100, ES-610, EHR-O, TS408. EHR-0832-O, 8, 32, 100, ES-610, EHR-O, TS408. 於 echaintool.com -
#35.Electronic Health Record | Indian Health Service (IHS)
The Electronic Health Record (EHR) website is designed primarily for IHS, Tribal, and Urban (I/T/U) Indian health care facilities that are actively involved ... 於 www.ihs.gov -
#36.What's an EHR? | eHealth Ontario | It's Working For You
An electronic health record (EHR) is a secure lifetime record of your health history. It gives your health care team, including family doctor, nurses, ... 於 ehealthontario.on.ca -
#37.Electronic Health Record Systems: Definitions, Evidence, and ...
Electronic health record (EHR) systems are increasingly considered to be one of the most relevant information technology tools for strengthening health ... 於 publications.iadb.org -
#38.Whats in my EHR and who can access it? - HealthChampion
An EHR, or electronic health record, is a digital compilation of your health information. EHR includes your EMR – plus many other ... 於 myhealthchampion.com -
#39.What is EHR: Benefits and Disadvantages - NIX United
EHR or Electronic Health Record is a patient's medical history stored in digital format. Implementing and meaningful use of EHR systems improves patient ... 於 nix-united.com -
#40.ASUS - 人才招募
華碩整合了不同領域的優秀人才,建立全方位的工作團隊,也讓同仁在充滿活力的情境下發揮想像力與創造力,我們積極培育世界級的產品創新研發及品牌行銷人才。 於 ehr.asus.com -
#41.EHR Software - Cloud & Al-Based Electronic Health Records
talkEHR is a MU3 certified, virtual assistant, cloud & artificial intelligence (AI) based electronic health records (EHR) software that understands you. 於 www.talkehr.com -
#42.EHR, Electronic Health Record - Comarch
Comarch EHR is an IT system designed for viewing electronic documentation in all types of medical facilities. 於 www.comarch.com -
#43.EHR-2 JST Sales America Inc. | 連接器、互連元件| DigiKey
今日訂購,今日出貨。JST Sales America Inc. 的EHR-2 – 2 矩形連接器- 外罩插孔天然色0.098" (2.50mm)。Digi-Key Electronics 提供數百萬款電子元件的價格及供貨 ... 於 www.digikey.tw -
#44.[1706.03446] Deep EHR: A Survey of Recent Advances in ...
The past decade has seen an explosion in the amount of digital information stored in electronic health records (EHR). While primarily designed ... 於 arxiv.org -
#45.Effective Reporting Could Improve Safe Use of Electronic ...
Despite the near ubiquitous adoption of electronic health record (EHR) systems to replace paper files in hospitals and doctors' offices ... 於 www.pewtrusts.org -
#46.Country has national Electronic Health Record (EHR)
Electronic health records (EHRs) are real-time, patient-centred records that provide immediate and secure information to authorised users. EHRs typically ... 於 www.who.int -
#47.EHR SERIES-Hitano Enterprise Corp. 奇彥企業有限公司
EHR SERIES. Introduction. High Temperature Radial Type,105℃, 2000 hours assured Load Life. Applications: Communications equipment, switching regulators, ... 於 www.hitano.com.tw -
#48.MEDITECH EHR Software | MEDITECH
Today, more than 2300 institutions worldwide use MEDITECH's fully integrated, interoperable EHR software to provide healthcare to the communities they ... 於 ehr.meditech.com -
#49.Electronic Health Records | CMS
EHRs are the next step in the continued progress of healthcare that can strengthen the relationship between patients and clinicians. The data, ... 於 www.cms.gov -
#50.Electronic Health Records (for Teens) - Nemours KidsHealth
An EHR is a computerized collection of a patient's health records. EHRs include information like your age, gender, ethnicity, health history, medicines, ... 於 kidshealth.org -
#51.eHR電子報 - 叡揚資訊
GSS叡揚資訊成立於1987,是台灣資訊軟體業的領導廠商,也是區域級資訊軟體與雲端SaaS服務供應商。長期關心客戶的經營需求,經由成熟的軟體工程、先進的協同、行動 ... 於 www.gss.com.tw -
#52.Cloud-Based EHR Software - SaaS Patient Record ... - RXNT
RXNT's ONC-HIT-certified EHR/EMR Electronic Medical Records software supports any practice. Perfect for patient scheduling, lab orders and more. 於 www.rxnt.com -
#53.eHealth - Home
Joining the Electronic Health Record Sharing System (eHealth) Lifelong eHR for you and your family ... Electronic records to enable authorised healthcare ... 於 www.ehealth.gov.hk -
#54.eHR Viewer - eHealth Saskatchewan
The electronic Health Record (eHR) Viewer is a secure website authorized health care providers can use to access patient information, no matter where a patient ... 於 www.ehealthsask.ca -
#55.The 10 Most Common Inpatient EHR Systems by 2021 Market ...
Vendors Epic and Cerner collectively hold about 55 percent of the inpatient EHR market, but proprietary systems such as Medhost are holding their own. 於 www.definitivehc.com -
#56.JST (JAPAN SOLDERLESS TERMINALS) EHR-3
購買EHR-3 - Jst (japan Solderless Terminals) - Connector Housing, EH, Receptacle, 3 Ways, 2.5 mm, EH Series Contacts。e络盟提供優惠價格、當日出貨、快速 ... 於 tw.element14.com -
#57.Implementing Measurement Science for Electronic Health ...
How did it happen that the electronic health record (EHR) emerged as a concern regarding clinicians' well-being, and what is the path ... 於 jamanetwork.com -
#58.Practice Fusion: Cloud Based EHR - Electronic Health Records
'Deliver better care more efficiently with the #1 electronic health record. Our industry-leading EHR was developed with feedback from over 150000 ... 於 www.practicefusion.com -
#59.EHR Go - Real-world experience for all areas of your curriculum
Go is an educational Electronic Health Record (EHR) and learning platform used in all healthcare disciplines with customizable patient cases ... 於 ehrgo.com -
#60.Data Note: Public's Experiences With Electronic Health Records
At the beginning of the health care reform debate in 2009, KFF polls showed the public held mixed views of EHRs. Most said it would improve ... 於 www.kff.org -
#61.Electronic Health Records by CompuGroup Medical - cgm.com
Also known as an electronic medical record, or EMR, an electronic health record improves a healthcare practice's efficiency and access to patient data by ... 於 www.cgm.com -
#62.Electronic Health Records (EHR) Incentive Program
Electronic Health Records (EHR) Incentive Program. Important Program Deadlines. For the final 2021 program year, the application submission ... 於 eohhs.ri.gov -
#63.EHR/EMR Analysis and Insights - HIT Consultant
HIT Consultant offers the latest in Healthcare Technology news, updates & trends. Get the latest updates on EMR News and EHR News here. 於 hitconsultant.net -
#64.使用Teams 虛擬就診- 整合至EHR - Microsoft Docs
Microsoft TeamsEHR (EHR) 連接器的電子健康記錄功能,讓臨床醫師可以直接從EHR 系統在Teams 中啟動虛擬病患拜訪或諮詢。 Microsoft Teams 建立 ... 於 docs.microsoft.com -
#65.DrChrono: EHR, Practice Management, Medical Billing, & RCM
DrChrono's cloud based EHR, medical billing software and RCM services fully equip your practice and let you provide optimal patient care. 於 www.drchrono.com -
#66.What is an electronic health record (EHR)? | HealthIT.gov
An electronic health record (EHR) is a digital version of a patient's paper chart. EHRs are real-time, patient-centered records that make ... 於 www.healthit.gov -
#67.EHR Go | LinkedIn
EHR Go | 119 位LinkedIn 關注者。Go is an educational Electronic Health Record (EHR) and learning platform used in all healthcare disciplines. 於 tw.linkedin.com -
#68.EMR & EHR Software: Electronic Medical Record Service
Our EHR / EMR software unifies your practice to improve clinical care. Learn more about our electronic medical record / electronic health record services, ... 於 www.advancedmd.com -
#69.Electronic health records | Shaping Europe's digital future
The European Commission has adopted a Recommendation on a European electronic health record exchange format to unlock the flow of health data across ... 於 digital-strategy.ec.europa.eu -
#70.Electronic Health Records (EHR) - Webmedy
What is EHR? An electronic health record is a collection of secure, real-time electronically-stored health information records for a patient that make ... 於 webmedy.com -
#71.Electronic Health Records (EHR, EMR) | Healthcare IT News
A paper published in the Journal of the American Medical Informatics Association noted that EHRs often misclassify American Sign Language as an "other" ... 於 www.healthcareitnews.com -
#72.EHR - MŪSDIENĪGI MUZIKĀLI IZKLAIDĒJOŠI
Klausies EHR FM radio · Rīga 104,3 · Liepāja 96,1 · Daugavpils 92,9 · visas frekvences ... 於 www.ehrhiti.lv -
#73.Electronic Health Record (EHR) - eHealth Ireland
A national Electronic Health Record (EHR) has been identified by HSE National Directors and clinical leaders as a key capability requirement for the future ... 於 www.ehealthireland.ie -
#74.Children's Electronic Health Record Format
What is the Children's EHR Format? · Prenatal and newborn screening tests · Immunizations · Growth data Information for children with special health care needs ... 於 digital.ahrq.gov -
#75.MN EHR Consortium - Hennepin Healthcare
The mission of the MN EHR Consortium is to improve health by informing policy and practice through data-driven collaboration among members of Minnesota's ... 於 www.hennepinhealthcare.org -
#76.eHR:隨著醫療信息化行業的不斷探索 - 中文百科知識
隨著醫療信息化行業的不斷探索,EHR(Electronic Health Record)也逐漸被業界人士交流,這個EHR是電子健康檔案的縮寫。在衛生部的檔案中有對這個名詞的專門解釋。 於 www.easyatm.com.tw -
#77.Electronic health records (EHR) as a service
Program updates As we continue to move forward through the EHR implementation, you can look to this location to house updates and milestones ... 於 www.hca.wa.gov -
#78.EMR vs EHR - What is the Difference? | CareCloud
The EMR vs EHR battle has been constant for years. Yet the distinction between electronic medical records (EMR) and electronic health records (EHR) is still ... 於 www.carecloud.com -
#79.電子健康紀錄- 维基百科,自由的百科全书
電子健康紀錄,又称为电子健康档案,简称EHR (electronic health record),是電子化的個人健康紀錄(病歷、心電圖、醫療影像等),電子健康紀錄可以經由電腦或網路存 ... 於 zh.wikipedia.org -
#80.Epic | ...with the patient at the heart
Epic leader talks EHRs, and how they're helping with COVID-19 vaccination efforts ... Epic's EHR Optimization Mitigates SDOH, Promotes Care Coordination. 於 www.epic.com -
#81.Directorate for Education and Human Resources (EHR) | NSF
EHR supports excellence in U.S. STEM education at all levels, in all settings for the development of a diverse and well-prepared workforce of scientists, ... 於 beta.nsf.gov -
#82.FastStats - Electronic Medical Records - CDC
Percent of office-based physicians using any EMR/EHR system: 89.9% ... Source: 2019 National Electronic Health Records Survey Public Use File National ... 於 www.cdc.gov -
#83.Better EHR Book - NCCD - UTHealth School of Biomedical ...
"Better EHR: Usability, Workflow and Cognitive Support in Electronic Health Records" -- a book published by the National Center for Cognitive Informatics ... 於 sbmi.uth.edu -
#84.Package EHR - CRAN
Process and analyze electronic health record (EHR) data. The 'EHR' package provides modules to perform diverse medication-related studies using ... 於 cran.r-project.org -
#85.Meaningful Use: Electronic Health Record (EHR) incentive ...
Get more information on the incentives Medicare and Medicaid provide for medical practices to adopt new records, billing and practice management procedures. 於 www.ama-assn.org -
#86.What is Electronic Health Record (EHR) | IGI Global
Included in the EHR are progress notes, problems, medications, vital signs, patient demographics, past medical history, immunizations, and laboratory ... 於 www.igi-global.com -
#87.New York Medicaid Electronic Health Records (EHR ...
New York Medicaid Electronic Health Records (EHR) Incentive Program. Through the NY Medicaid EHR Incentive Program, eligible professionals (EPs) and ... 於 www.health.ny.gov -
#88.Optum EHR Data for Life Sciences
See the bigger picture of patient health care journeys. Optum electronic health record (EHR) data provide valuable context to ignite insights and fuel ... 於 www.optum.com -
#89.EHR Software - Electronic Medical Records | athenahealth
A cloud-based scalable EHR that intuitively organizes the patient visit, helps providers document faster, and sets your organization up for success. 於 www.athenahealth.com -
#90.EHR Systems: Key Features, Capabilities, and Adoption Tips
Electronic Health Record (EHR) systems have become the golden standard for the aggregation, storage, and exchange of medical data. 於 easternpeak.com -
#91.openEHR Home
openEHR provides open source specifications and reference implementations of future proof EHR systems. 於 www.openehr.org -
#92.Electronic Health Record - an overview | ScienceDirect Topics
Electronic health records (EHR) refers to the digital records of patients. From: Handbook of Data Science Approaches for Biomedical Engineering, 2020. Related ... 於 www.sciencedirect.com -
#93.EHR Systems - American Academy of Ophthalmology
We are working with each EHR system vendor and each practice to connect the EHR data with the IRIS Registry and configure the data connections and mappings ... 於 www.aao.org -
#94.EMR vs EHR: Understanding Electronic Health & Medical ...
If an EMR is a snapshot of a patient's medical history, the Electronic Health Record system is the whole movie. EHRs are reliant on EMRs and are essentially ... 於 www.experityhealth.com -
#95.EHR-032-064 - 曜宇科技股份有限公司
首頁; EHR-032-064. 自動化光學 · CAD-同軸擴散光源 · EDL-高亮度棒狀光源 · DAL-可調角度棒狀光源 · DOM-半球形擴散光源 · HSC,NSC-內同軸光源 · EHR,EPR,ELR,SLR ... 於 www.exlite.com -
#96.Developing Recommended Practices For Analyzing EHR ...
Researchers are creating a set of recommended practices to use when analyzing electronic health record databases, improving patient ... 於 healthitanalytics.com -
#97.Electronic Health Records (EHR) and Interoperability - IRIS ...
Electronic Health Records (EHR) and Interoperability: Understanding two key concepts for a better Public Health response · View/Open · Date. 於 iris.paho.org -
#98.Electronic Health Record Information | NC HIEA
Electronic Health Record Information ... The NC HealthConnex technology and tools provide near real-time, comprehensive clinical data about your patients. You ... 於 hiea.nc.gov